Explore the features of Discover and become an expert survey creator!
Discover Help Guide
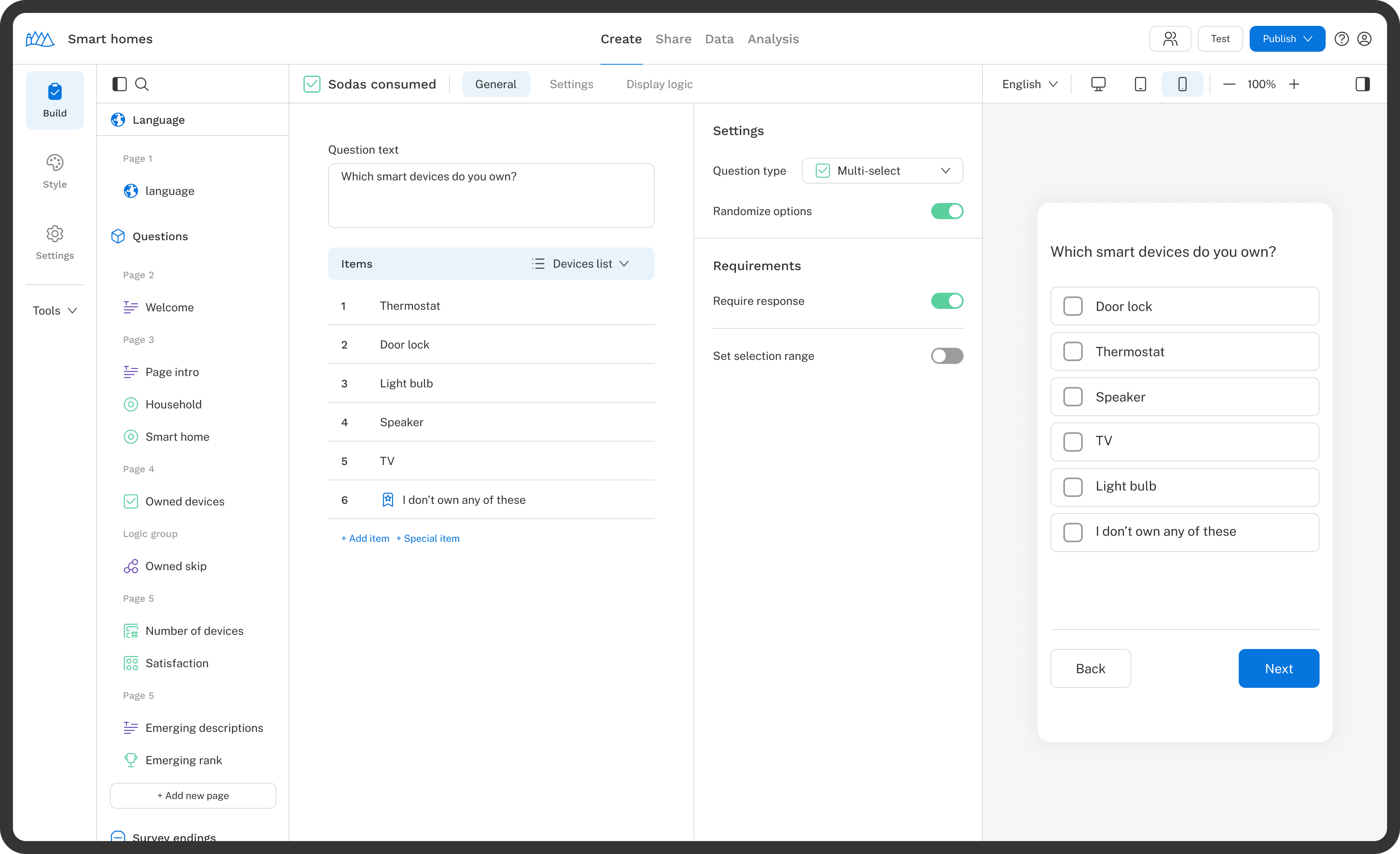
Explore the features of Discover and become an expert survey creator!
Support: support@sawtoothsoftware.com
Consulting: analytics@sawtoothsoftware.com
Sales: sales@sawtoothsoftware.com